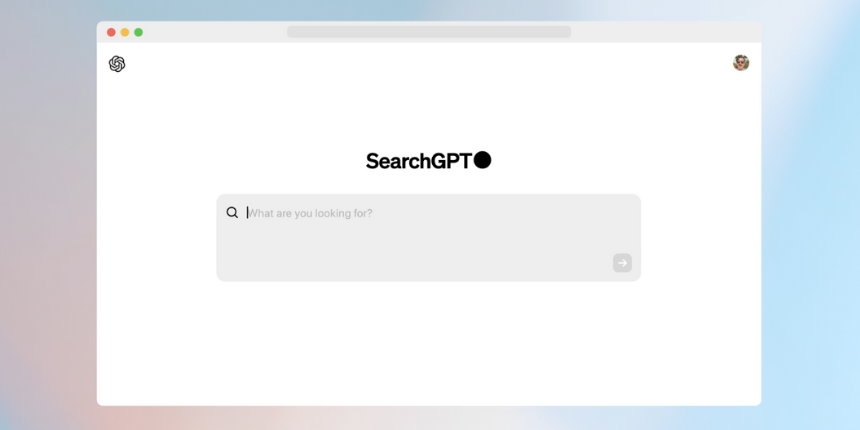
Navigate an unpredictable landscape with actionable, data-driven strategies tailored for your business from the brand down to the local level.
The latest Large Language Models (LLM), although toddlers, are in many ways much smarter than the average human is—or will ever be. Not only do they know more, but they have a much greater capacity to process and act on vast arrays of instructions.
We’ve recently explored the potential of LLMs to interpret or generate text considering numerous parameters with extraordinary precision. We have demonstrated their ability to evaluate unstructured text blocks and grade them along various scales to great success. Additionally, we have been able to generate specific, tailored content using a complex series of instructions that can be tuned with precision to the same set of scales.
We are entering a new era of performance-based, data-driven content where we can transform LLMs into written content production facilities, complete with a control board of knobs and dials to numerically fine-tune optimal marketing content across various formats.
Here, we share our initial experiment findings and offer a general methodology to harness LLMs to extract quantitative measures from otherwise unstructured text, opening the door to statistical analysis and optimization of content creation.
Source: Analytics Vidhya
Large language models are constructed using mathematical vectors. This foundation allows them to adeptly translate blocks of text—rating, scoring, or mapping content into numeric values that capture the distinct qualities of each block. For example, we can ask the model to evaluate how ‘technical’ this blog is on a scale of 1-10, where the boundaries are defined by example or by user instruction. Similarly, LLMs can reverse this direction to generate blocks of text that correspond to numeric scores of a certain attribute submitted by a user. For example, you can ask the LLM to write a blog post with the degree of technicality scoring 9 out of 10.
We can additively include more concepts to build a conceptual space. This is what we term a conceptual Cartesian space, which the LLM can refer to for content generation. We can plot a point in this space to define an idea based on its position relative to each of the axes that define our space.
Source: https://serokell.io/blog/language-models-behind-chatgpt
Subscribe to our monthly newsletter.
We conducted a series of experiments to validate the effectiveness and flexibility of conceptual Cartesian mapping using LLMs. We took a ‘ground-up’ approach to validate our methodology, starting with basic experiments and increasing the complexity at each step.
This experiment explores the LLM’s ability to scale content along linear gradients, providing users with control in generating or evaluating text. We examined different scale ranges (1-10, 1-100) and demonstrated the model’s adherence to specific scoring frameworks. Results affirm the model’s ability to methodically follow chosen gradients.
In this experiment, we tested the influence of alternative scoring methods on text output. The LLM is instructed to apply various scoring frameworks, showcasing the model’s adaptability. It successfully crafts responses based on specific rules, indicating the customization potential of LLMs for diverse applications. We even used a respected psychological framework to grade (or diagnose) empathy scores for a block of content.
This experiment delves into the model’s performance in multi-dimensional spaces. The study introduces concepts like practicality and technicality as additional axes, illustrating the LLM’s ability to handle complex ideas and multiple dimensions effectively. The results indicate the model’s agility in navigating intricate multi-dimensional spaces.
This experiment explores the LLM’s capability to quantitatively analyze ideas relative to other ideas, not gradients along a single axis. One significant practical application for marketers is positioning content relative to competitors; we got the LLM to generate housing policy for a fictitious mayoral candidate that is quantitatively positioned relative to several existing candidates.
Our study demonstrated the model’s ability to handle open content generation tasks with quantitative precision, showcasing its potential in environments where ideas lack strict predefined frameworks.
If we bind the conceptual Cartesian position of content with traditional metrics, we can analyze the performance of published content against newly available numeric values. For example, we can study the social media posts (i.e., likes, shares, click through rates) against conceptual scores assigned by the model for attributes like humor, empathy, and technicality. Through statistical analysis, we can identify the optimal mix of each conceptual attribute for a given context and use that co-ordinate position to generate new content for enhanced performance.
The innovative combination of conceptual Cartesian mapping and LLMs gives us a new, methodical, precise approach to general content creation.
Businesses can tailor their messaging with precision, ensuring maximum engagement with their target audience whilst positioning their content relative to competitors. Political campaigns can craft nuanced narratives relative to other candidates or polling results. Educational institutions can create customized learning materials, enhancing student engagement and comprehension at the individual level.
Want to be ready for that future? Talk to a DAC strategist today!
Navigate an unpredictable landscape with actionable, data-driven strategies tailored for your business from the brand down to the local level.
Navigate an unpredictable landscape with actionable, data-driven strategies tailored for your business from the brand down to the local level.
Navigate an unpredictable landscape with actionable, data-driven strategies tailored for your business from the brand down to the local level.
Subscribe to our monthly newsletter.