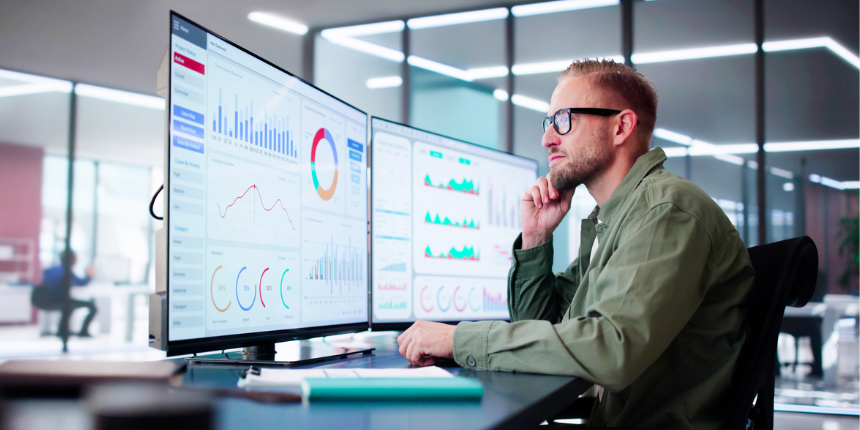
Unleash the true value of your data with our center of excellence, Proove Intelligence.
Last week I went to the Data Driven Business conferences, which are essentially eMetrics, Conversion Conference, and Predictive Analytics World all at the same venue in London.
In terms of analytics maturity, I would say that eMetrics was more intended to deliver the business value of data analytics to a wide audience, Conversion Conference was more focused on discovering particular tools and techniques for analytics processes, and Predictive Analytics world was the highest maturity stage (so far) in the art and science of data analytics.
Or, in other words, a bunch of introverts from all over the globe who are quiet and friendly at the lunch queue but would speak loudly and boldly about their grains of data enlightenment. In terms of personas, corresponding target audiences would look like this:
eMetrics: anxious beginners & relaxed experts
Conversion Conference: on-site conversion tacticians
Predictive Analytics World: VP, CEO, CFO, Head of Data / Analytics / BI
Whilst constantly absorbing the knowledge from all three domains of data and analytics (web analytics, UX & conversion rate optimization and predictive analytics), I have come up with this business process of data & analytics (click any to skip straight there):
First comes problem recognition. It requires as much effort as any other stage if not more. You need to ask the right question. Full stop.
Here the debate as to what is more important, domain knowledge or data science skills, is not relevant. Domain knowledge is the king when you need to ask the right question. We need to sit down with a client or, even better, spend a few days together and drill down as deep as needed to reveal the initial source of the pain.
What if you skip through the problem recognition stage and come up with a wrong question? The cost of error will be as much as the time spent coming to realise that the question was wrong. Doing what matters is called efficiency and a seemingly ‘easy’ job is usually a trap.
For more, check out Dean Abbot’s retrospective but not less valuable speech about his Five pet peeves of predictive analytics.
Please refer to the next presentations for a high-level view on the value of data driven business:
With the right question in mind we can approach the data collection and all of the tagging implementation.
Who has GA (or Kissmetrics or Adobe Analytics or whatever else you prefer for data collection from user cookies) implemented on their website? Every business.
Who has tag management system and nicely organized data layer in place instead of heavy on-page tags implementation? Only some.
Who has agile business processes to keep their website and tag management system clean of redundant tags, employs clear measurement hierarchy, and updates and improves their dozens of tags without creating a project lasting a couple of months? A rare breed of business which uses data as a competitive advantage.
Only the domain experts can really tell you what their major business metrics are. But accurate data collection, which could seem to be boring and hugely time consuming, could solve most of the problems around decisions being based on erroneous data.
Considering the principle “I am what I eat”, data collection is responsible for the physical health of all of your decisions. If you feed the model with the wrong / erroneous / incomplete data, it’s going to give you wrong / erroneous / incomplete answers to your questions.
Sorry, there’s no magic to fix this. Science needs clean data.
I’ve been following the blog of Simo Ahava for a few years now and he is one of few creative JS developers who is not afraid to share his knowledge. He had a great presentation at eMetrics and Conversion Conference, but his blog posts speak for themselves.
I also have an additional bookmark for event tracking ecosystem by Snowplow and the book called “DOM enlightenment” in my Amazon cart after Simo’s Q&A today at the conference.
If “analysis” can be interpreted as the process of learning the unknown, this analysis can be of three main kinds:
This includes a thorough investigation of current market conditions and better understanding of the business needs and constraints. By changing the scope of the analysis from high-level market view to the granular data, analysts create a big picture with drill-downs for anticipated market threats and opportunities.
It’s important to remember that the market is not static and the conditions such as seasonality, customer demand and competitors activity are changing all the time. Monitoring these changes is important, but much more important is to react to those changes on time.
Obviously, the initial research of digital markets is focused on a global picture which works for a long time whilst market monitoring is relevant to the current situation and requires immediate reaction to the threat and emerging opportunities.
What data does your business already have? Start with this question and identify the areas where you want to drill down.
What do you know about your customers? Summarize your knowledge and find the gaps.
What are you good at? Your business is unique and there definitely should be the areas where you are more successful than your competitors. Focus on those strengths and enhance them even more with data-driven power.
Whilst the whole data industry is preoccupied with problem-solving and catching up with their competitors, you should start by enhancing your strengths for quick wins. Gain your first results, evaluate and improve. Desk research does not create value, the value is created from testing the analysts’ recommendations from that research and choosing the most efficient ones to scale up for the whole business.
Subscribe to our monthly newsletter.
If you don’t know where to start, as a “what-if” question. Find something which you have doubts about and set up a small experiment to find the answer in data. It’s much cheaper to come to a strategic improvement following a set of specific iterative experiments than to make a big change and fail. Learn how to fail quickly and analyse your performance before your competitors do.
Key takeaways
I took five most important takeaways for myself in data analysis (and I am not talking statistics here):
Check out these presentations for some practical tips and tricks:
To reiterate: every assumption should be tested in practice. I thought the small presentation hall of Predictive Analytics World would only attract geeks like me with degrees in Statistics, Maths and Operational research. Surprisingly, however, there was a concentration of senior managers, including one finance director speaking about the effective allocation of marketing budget due to marketing mix attribution. It seems there are quite a few others who prefer data modelling to a crystal ball.
We all want to be a little bit better every day. We want to do our job better. We want to be helpful and feel important. When this all is projected to business it mostly means to be efficient in short-term and effective in long-term.
Marketing is essential for business development. Can machine learning be applied to marketing to decrease inventory, reduce the risk of a failure, increase profit margins and reach high operational efficiency, inform strategic decisions, or improve business performance?
Brands like Expedia, Shell, Barclays, Argos and Sainsbury’s were already sharing their progression in predictive data modelling for greater business efficiency.
Without getting into too much details in the specifics of different algorithms I can break the most interesting case studies of improving business efficiency from this Predictive Analytics World into three main groups:
Everybody in the offline business understands the costs that come with excess or insufficient inventory. If your business under-produces, you can’t serve the demand and your competitors easily take your market share. If you overproduce, you are paying more for storage. You want to produce “just enough” to keep the inventory low.
But can the same analogy be applied to digital marketing?
If your marketing budget is low only a fraction of your target audience can see your ads and have a chance to click on them. If your budget is much higher than the existing demand you will need to find new audiences to engage, and your efficiency in conversion rate and revenue per visitor is likely to decrease.
So, what would be “just enough” in digital marketing? How to measure it, monitor the changes, and predict the demand? Please feel free to have a look at the next presentations for some answers and practical ideas for data modelling.
Which customers are more likely to be ready for up-sell? Which product groups are likely to perform best with regards to each other? Which channels better perform as closers, holders or initiators of the lead? How can you predict the value of the lead based on the sequence of marketing channels? Check out the next presentations for cutting-edge models in classification algorithms.
They say correlation does not imply causation. But what if you need to see the causation? What’s the most important factor for customers in making purchase decision, buying from your company rather from your competitors? Why does one advert perform better than another one? How do you make analysis granular and optimize on-the-go?
Check out my favourite thought leader in predictive analytics for some insight on data modelling in behavioural / contextual marketing.
[av_hr class=’short’ height=’50’ shadow=’no-shadow’ position=’center’ custom_border=’av-border-thin’ custom_width=’50px’ custom_border_color=” custom_margin_top=’30px’ custom_margin_bottom=’30px’ icon_select=’yes’ custom_icon_color=” icon=’ue808′ font=’entypo-fontello’]
If you are seriously interested in applied predictive data analytics, please refer to Dean Abbott and see what he shares with the industry from his work. And even if you can’t be bothered with data modelling yet, you can have a look at his recorded speeches to see where this big Analytics ship is turning to.
Personally as an analyst I may know lots about data science, customer intelligence, cross-industry best practices, and so on, but it does not have any value until applied to a client’s business. Therefore, my best learning curve should certainly be listening to clients’ points of view, concerns and complaints. If a client speaks up about their concerns I might have a relevant solution based on my knowledge and experience.
Here are just a few interesting quotes from the clients presenting their own work and ongoing results at eMetrics / Conversion optimisation and Predictive Analytics:
“Don’t tell me that it is easy when it is not. Everything has a cost and a budget”
“I don’t care about sunshine and rainbows in your case studies when you cannot make the change for my own business”
“Anybody in the team should have the opportunity to fit in your solution”
Overall, I see that big data:
If you think predictive analytics’ shoes are too big for you, start with bite-sized improvement, start with what is relevant to you. Take an analyst in your team. Succeed and scale up the amplification effect. Or fail quickly and revise your assumptions.
And as an analyst I always do my best in order to:
If you’d like to talk to me about your own analytics, get in touch or ask your question here.
Unleash the true value of your data with our center of excellence, Proove Intelligence.
Unleash the true value of your data with our center of excellence, Proove Intelligence.
Unleash the true value of your data with our center of excellence, Proove Intelligence.
Subscribe to our monthly newsletter.